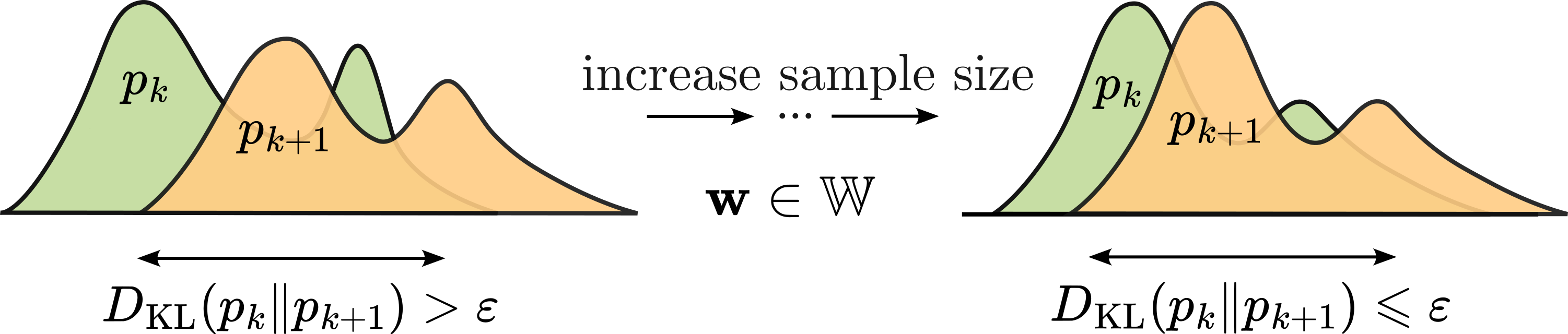
Sample Size Determination: Posterior Distributions Proximity
This paper presents two approaches to determining a sufficient sample size based on the proximity of posterior distributions of model parameters on similar subsets.
This paper presents two approaches to determining a sufficient sample size based on the proximity of posterior distributions of model parameters on similar subsets.
This paper introduces a method for estimating the Hessian matrix norm in convolutional neural networks, offering insights into the loss landscape’s local behaviour, supported by empirical convergence analysis.
This paper propose a method for creating a linear model that predicts changes in fMRI signals based on video sequence images. A linear model is constructed for each individual voxel in the fMRI image, assuming that the image sequence follows a Markov property.
This paper presents two approaches to determining a sufficient sample size based on likelihood values from resampled subsets.
This paper explore the convergence of the loss landscape in neural networks as the sample size increases, focusing on the Hessian matrix to understand the local geometry of the loss function.
The method proposed is the first linearly convergent first-order decentralized algorithm for problems with general affine coupled constraints.